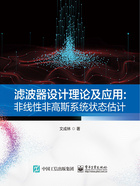
2.5 特征函数及其基本性质
本节主要介绍特征函数的定义、特征函数的性质、常用概率密度函数的特征函数、特征函数的应用、中心极限定理、利用特征函数求估计量的概率密度函数等。
2.5.1 一维特征函数
1.特征函数的定义
(1)离散随机序列的特征函数的定义:设一维离散随机序列x(k)∈R1的离散事件概率为P{X=x(k)}=pk,则该离散随机序列的特征函数为

(2.5.1)
(2)连续随机变量的特征函数的定义:设一维随机变量x∈R1的概率密度函数为f(x)∈R1,则该随机变量的特征函数φx(k) 定义为核函数eikx的期望值。

(2.5.2)
随机变量x的特征函数的本质是随机变量x的概率密度函数f(x) 的Fourier变换。任何概率密度函数都存在特征函数。
例2.5.1 设随机变量X服从退化分布,即

求该随机变量X的特征函数。
由离散随机序列的特征函数的定义

有

(2.5.3)
例2.5.2 设随机变量X服从参数为p的0-1分布(两点分布),求其特征函数。
由

可得

(2.5.4)
例2.5.3 设随机变量X服从参数为n,p的二项分布,求其特征函数。
由离散随机序列特征函数的定义可得

(2.5.5)
例2.5.4 设随机变量X服从参数为λ的泊松分布,求其特征函数。
由离散随机序列特征函数的定义可得

(2.5.6)
例2.5.5 设随机变量X服从[−a,a] 均匀分布,求其特征函数。
服从[−a,a] 均匀分布的随机变量的概率密度函数为

根据连续随机变量特征函数的定义,当t≠0 时,有

(2.5.7)
当t=0 时

例2.5.6 设随机变量X服从参数为λ的指数分布,求其特征函数。
根据连续随机变量特征函数的定义,有

(2.5.8)
2.特征函数与概率密度函数的关系
已知一维随机变量x的概率密度函数f(x) ,人们往往关心其特征值(如均值、方差)。特征值提供了概率密度函数最重要的信息,但不能确定概率密度函数的所有性质。特征函数与概率密度函数是一一对应的。概率密度函数由特征函数的反Fourier变换唯一确定。

(2.5.9)
也就是说,概率密度函数f(x) 与其特征函数φx(k) 是等价的。
3.特征函数的性质及证明
1)特征函数的性质
(1)φx(0)=1
(2)|φx(k)|≤1
(3)
(4)若y=ax+b,其中a,b为常数,则

(5)独立随机变量和的特征函数为各变量特征函数的积,即设x,y独立,则

(6)若E{xl} 存在,则φx(t) 为l次可导,并且对1≤m≤l,有

2)部分特征函数性质的证明
性质(5):独立随机变量和的特征函数为各变量特征函数的积,即设x,y独立,则

(2.5.10)
证明:由于x与y之间是相互独立的,因此,eikx与eiky之间也是相互独立的,从而有

(2.5.11)
可以推广到n个独立随机变量之和z=x1+x2+⋯+xn的特征函数,即

(2.5.12)
本性质(5)证毕。
利用反Fourier变换可求出随机变量z的概率密度函数:

(2.5.13)
性质(6):若E{xl} 存在,则φx(t) 为l次可导,并且对1≤m≤l,有

(2.5.14)
证明:因为E{xl} 存在,所以有。
于是含参数变量k的广义积分

(2.5.15)
可以对m求l次导数。
所以,对0≤m≤l,有

(2.5.16)
令k=0 ,即可得到

本性质(6)证毕。
4.常用概率密度函数的特征函数(表2.5.1)
表2.5.1 常用概率密度函数的特征函数

5.特征函数的应用
既然概率密度函数与特征函数是一一对应的,为什么还要引入特征函数呢?这是因为很多问题直接用概率密度函数不易处理,而利用特征函数进行处理就非常方便。
1)求均值和方差(以高斯分布为例)
设正态随机变量x的特征函数为

(2.5.17)
(1)求取均值。

(2.5.18)
(2)求取方差。

(2.5.19)
类似地,可以很容易求出各阶中心矩。
2)求概率密度函数的极限(以二项分布为例)
特征函数为

(2.5.20)
取极限p→0和N→∞,ν=pN为常数。

(2.5.21)
即二项分布在实验次数很多且均值保持不变时,趋向于泊松分布。同样可以证明ν很大时,泊松分布趋向于高斯分布。
3)求独立随机变量之和的概率密度函数
假设有两个独立的高斯随机变量x和y,均值为μx和μy,方差为和
,则z=x+y的特征函数为

(2.5.22)
这正是均值μz=μx+μy,方差的高斯分布的特征函数。
同样可证泊松变量之和仍服从泊松分布。
4)中心极限定理
定理2.5.1:假设有n个独立随机变量xj,均值与方差分别为μj和。在大n极限下,
为高斯随机变量,均值和方差分别为
和
。
证明:定义

则有

将yj的特征函数ϕj(k) 进行泰勒展开:

(2.5.23)
在大n极限下,忽略高阶项,可得

(2.5.24)
再定义

则z′的特征函数为

(2.5.25)
即z′为均值为0,方差为σ2/n的高斯分布。变换回,则z为均值为
,方差为σ2的高斯分布。
n有限时,中心极限定理成立的条件是每个xj的贡献都很小,即z由大量微小贡献组合而成。
例如,很多地方经常用12个(0,1]均匀分布的随机变量之和来近似高斯分布。

(2.5.26)
如果某个或某几个xi的贡献非常大,则求和的结果将明显偏离高斯分布。
5)求估计量的概率密度函数
(1)以指数分布

(2.5.27)
为例。其参数ξ的最大似然估计量为

其分布可以用特征函数法求得。
由于ϕx(k)=1/(1−ikξ) ,所以

(2.5.28)
的特征函数为

通过反Fourier变换可得到z的概率密度函数:

(2.5.29)

(2.5.30)
这是伽马分布,在n很大时趋向于高斯分布。
(2)要求取寿命的平均值,可以采用如下方式:

(2.5.31)
也可以利用概率密度函数进行积分来求取:

(2.5.32)
(3)对于λ=1/τ的最大似然估计量,求取其期待值:

可以先求的分布函数:

(2.5.33)
再用该函数求取期待值:

(2.5.34)
可以看出不是无偏估计量。
(4)求估计量期待值的置信区间。利用特征函数法求出估计量(如)的概率密度函数。有了估计量的概率密度函数(如
),很多问题都可以方便地进行处理,如求取置信区间。
对于给定的α,β以及观测值,通过

(2.5.35)

(2.5.36)
求得置信区间[a,b]。
2.5.2 二维随机变量的特征函数
1.定义
连续型:设(X,Y)是一个二维随机变量,其分布函数为F(x,y) ,x,y∈R1t1,t2为任意实数,记

(2.5.37)
称φ(t1,t2) 为(X,Y)的特征函数。
离散型:

(2.5.38)
其中,P(r,s)=P{X=r,Y=s} 。
设有n维随机变量

则称

(2.5.39)
为n维随机变量(X1,X2,⋯,Xn) 的特征函数。
2.二维随机变量特征函数的性质
性质1:设随机变量(X,Y) 的特征函数为φ(t1,t2) ,则有
(1)φ(0,0)=1 ,并且对任意t1,t2∈R,有|φ(t1,t2)|≤φ(0,0)=1 ;
(2);
(3)φ(t1,t2) 于实平面上一致连续;
(4)φ(t1,0)=φ(t1) ,φ(0,t2)=φ2(t2) 。其中,φ1(t1),φ2(t2) 分别为X和Y的特征函数。
性质2:设a1,a2,b1,b2皆为常数,(X,Y) 为二维随机变量,则随机变量(a1X+b1,a2Y+b2) 的特征函数为。
性质3:两个二元分布函数恒等的充分必要条件是它们的特征函数恒等。
性质4:设随机变量(X,Y) 的特征函数为φ(t1,t2),a1,a2,b为任意常数,则Z=a1X+ a2Y+b的特征函数为

(2.5.40)
定理2.5.2:随机变量(X,Y) 服从二维正态分布的充分必要条件是X与Y的任一线性组合
aX+bY+c
服从一维正态分布。其中,a,b,c为任意常数,且a,b不全为0。
3.相互独立随机变量的特征函数
定理2.5.3:n个随机变量相互独立的充分必要条件为(X1,X2,⋯,Xn) 的特征函数满足

(2.5.41)
推论2.5.1:设X1,X2,⋯,Xn为n个相互独立的随机变量,令,
,则Y和Z的特征函数分别为

(2.5.42)
注:对x,y∈Rn,也有类似描述。